Foodborne diseases are important global public health and food safety issues. In recent years, foodborne diseases have shown the characteristics of trans-regional spread, variability and unpredictability. Relying on the national key R&D project "Research on the Real-time Early Warning Technology System of Foodborne Diseases Based on Multi-source Data", the Big Data Department deeply integrates big data and machine learning technology with the actual needs of foodborne disease monitoring to achieve several research achievements in this cross-research field. The Big Data Department has published papers in the food science and technology journals Food Control and Foodborne Pathogens and Disease, as well as the medical information journal JMIR Medical Informatics.
Pathogenic bacteria are the main cause of foodborne diseases. Data mining and machine learning methods are used to mine the potential correlation between foodborne disease factors, so as to identify pathogenic bacteria and assist in the diagnosis and treatment of foodborne diseases. The research group proposed a method of using machine learning to identify pathogenic bacteria of foodborne diseases. The method extracted features from space, time, patient information, exposed food and so on, and used the features to build machine learning models, which realizes the identification of pathogenic bacteria of foodborne diseases and provides auxiliary support for the diagnosis and treatment of foodborne diseases. Further, aiming at the spatial-temporal prediction of the incidence of foodborne diseases, a spatial-temporal risk prediction model based on multi graph structured LSTM is proposed, which can construct a variety of spatial correlations and dynamically fuse. The structured LSTM model based on Encoder-Decoder is used to model the time dependence and space dependence of data at the same time, which realizes the multi-step prediction of disease risk (Fig. 1).
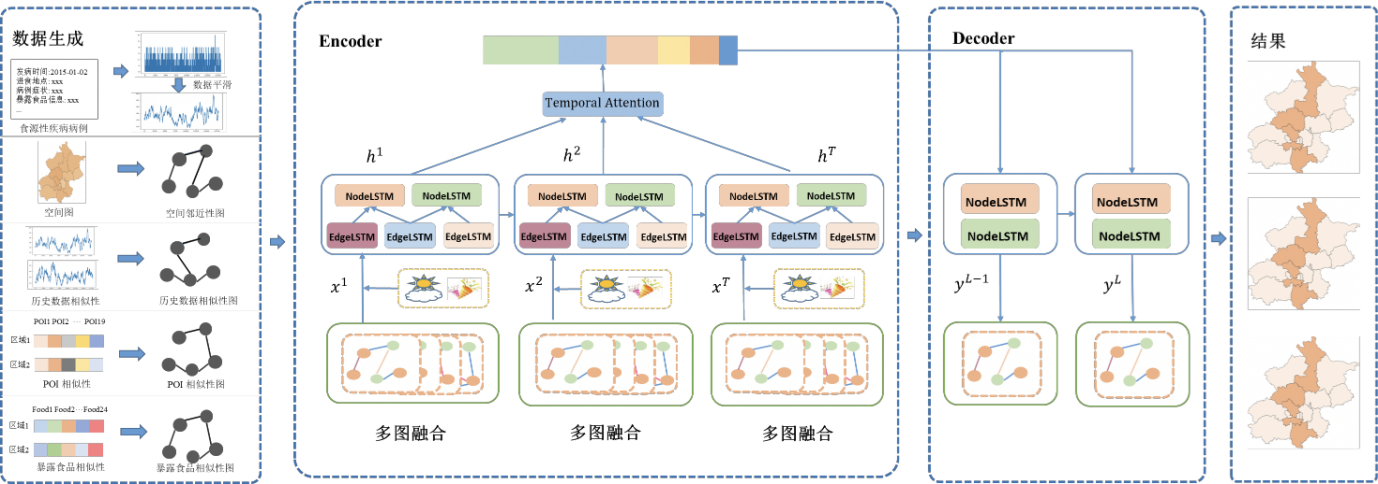
Figure 1:Spatio-temporal risk prediction model architecture for foodborne diseases
Foodborne disease outbreak refers to the occurrence of two or more foodborne disease cases with common exposure and similar symptoms. At present, the foodborne disease reporting and monitoring system obtains suspected foodborne disease outbreaks based on screening rules. However, this method generally has the problem of misjudgment. To further improve the accuracy of outbreak identification and prediction, the research group designed a foodborne disease outbreak identification model based on machine learning (Fig. 2). While identifying the outbreak, the effects of various characteristics and pathogenic factors on the discrimination results were analyzed , which can be used as a reference for medical workers.
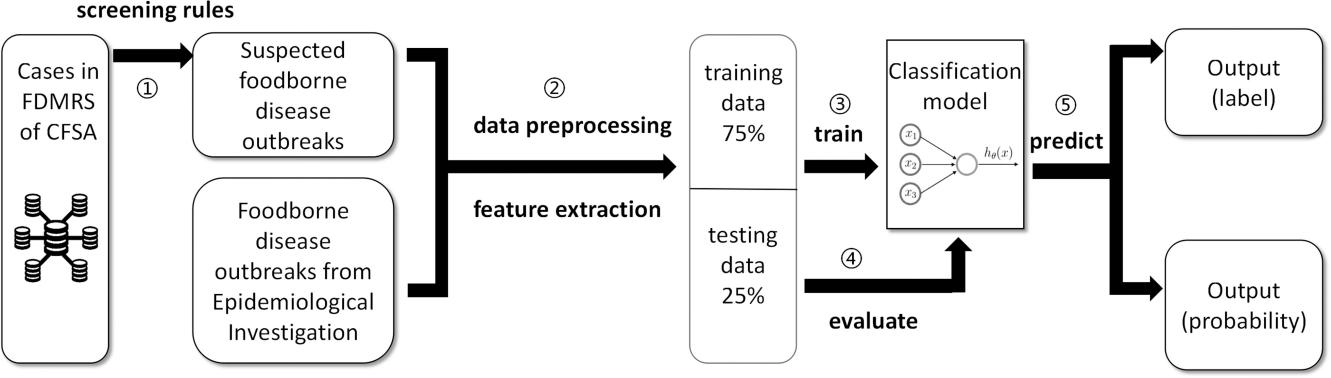
Figure 2:Optimization of foodborne disease outbreaks based on machine learning methods
Based on the above series of research results, the research group found that big data and machine learning technology can greatly improve the existing foodborne disease monitoring system in the stages of case reporting, disease diagnosis, outbreak identification and risk prediction. On this basis, the research group summarized the framework of foodborne disease monitoring system driven by machine learning. The framework will help to promote the intelligent improvement of foodborne disease surveillance system in the future.
For detailed information, please contact Professor. DU Yi (duyi@cnic.cn).