Under the guidance of Prof. Chi Xuebin, Prof. Wang Yangang and Prof. Wang Jue, Ph.D. candidate Yao Tiechui has recently published his academic results in the journal IEEE Transactions on Sustainable Energy and the journal Solar Energy.
Under the goal of peak carbon dioxide emission and carbon neutrality, the new generation of artificial intelligence technology provides new ideas and new means to promote the construction of a new power system with new energy as the mainstay. It has a wide range of application prospects in the distribution and consumption of new energy, energy conservation on the demand side, and regulation of power systems. The power output of photovoltaic(PV)systems is mainly affected by weather conditions. PV power stations need accurate weather data, especially solar irradiance, to predict their power output and thus improve the utilization of solar resources. On the one hand, accurate prediction of future power output using artificial intelligence techniques is essential to ensure the reliability and economy of power systems; on the other hand, publicly available datasets containing both power station and weather data can greatly facilitate the development of solar PV research.
The Artificial Intelligence Department proposes an intelligent forecasting framework for multi-source heterogeneous data and obtains satisfactory experimental results in the field of PV forecasting. The framework integrates the Advanced U-net model for processing unstructured data and the Encoder-decoder architecture for processing structured data. Based on the deep learning method, it can effectively extract and fuse the spatial and temporal features of various types of data (e.g., distributed multi-sensor real-time collected measurement data, numerical weather forecast and satellite image data), which effectively improves the accuracy of short-term irradiance prediction and further enhances the accuracy of PV power generation prediction. This technology is expected to be further implemented in the national grid dispatching demonstration application.
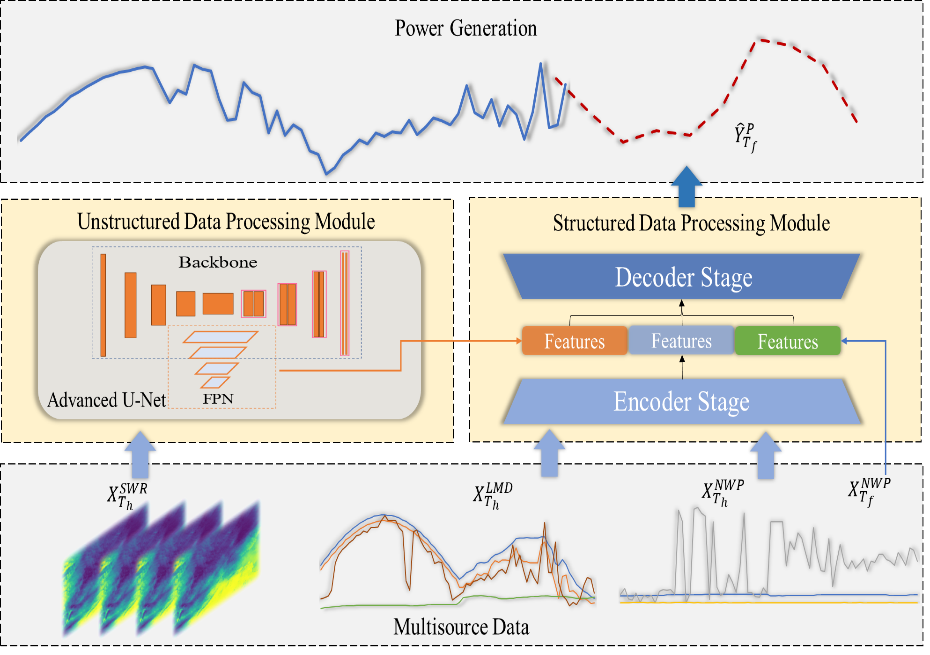
Figure 1Forecast framework for multi-source heterogeneous data
The Artificial Intelligence Department, together with the New Energy International College of Beijing Jiaotong University and State Grid Hebei Electric Power Co., Ltd., jointly releases a PV power output dataset with power station measurement data and meteorological data. The work collects the PV power output dataset and proposes pre-processing algorithms, and then releases a high-quality dataset of over 270,000 records and the corresponding Python toolkit. This work applies the clear-sky index Kpv and corresponding case studies to demonstrate the potential use of the dataset. The release of this dataset and toolkit play a catalytic role in the research of new energy meteorological applications such as solar energy prediction or performance assessment of PV system, etc.
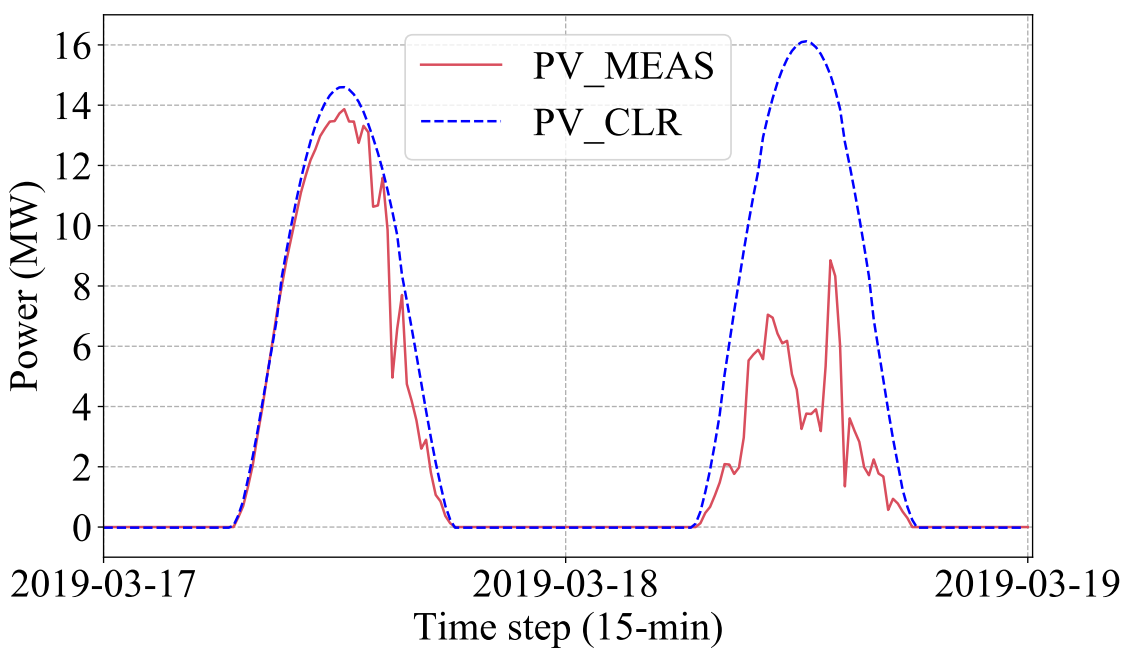
Figure 2 Schematic diagram of clear-sky index model modeling
For details, please contact Mr. Yao Tiechui (yaotiechui@cnic.cn).