TransMUSE: Research Progress on Traffic Prediction in Multi-Service Edge Networks
Date: Dec 23, 2022
Work-from-home pattern has put significant burdens on the management of broadband networks supporting multiple applications in recent years. Edge computing brings computation and data storage closer to the user to improve response times and save bandwidth. As edge computing evolves, a large amount of traffic is offloaded to the edge for processing, changing the flow of data in the network. The change of traffic flow poses new challenges to traffic prediction at the network edge, which can be used to optimize user experiences and reduce operational costs. 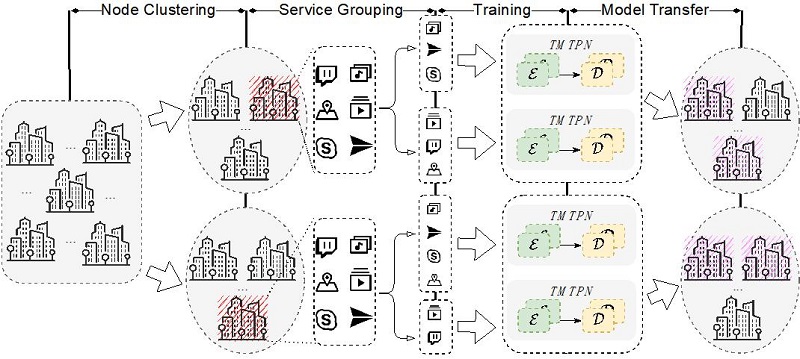
The intelligent traffic perception team at Advanced Network Department of CNIC conducts research on high-precision and fine-grained traffic prediction for intelligent network resource optimization based on edge nodes. The team uses real-world measurement to reveal that network traffic across geographical regions differs widely. Therefore models trained on historical traffic data observed in one region can hardly serve in making accurate predictions in other areas. Motivated to improve existed solutions limited by transerability, accuracy or cost-efficiency, the team proposed a multi-variable transferable network traffic prediction architecture called TransMUSE. TransMUSE improves the reusability of the prediction model, and reduces the huge overhead of maintaining separate models and the environmental footprint of developing multi-service edge networks. The team also proposed a time series clustering algorithm based on Wasserstein distance called WK-means, which is dedicated to improve the prediction performance, mine the association of time series with similar temporal patterns, and reduce the training data information entropy. The extensive experiments have been conducted based on the real-world dataset captured in Sichuan province. The average prediction performance on mean absolute error (MAE) metric decreases by 18% compared with the second best benchmark, and the accuracy loss on MAE caused by model migration is controlled within 2%. The research results have been published in Computer Networks.
For details, please contact XU Luyang (xuluyang@cnic.cn)
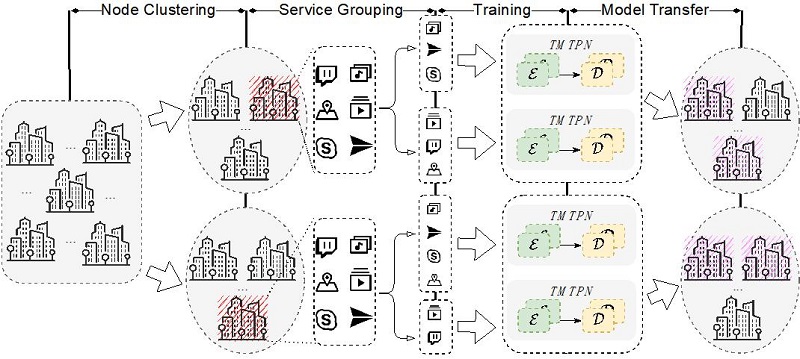
Figure: overall architecture of TransMUSE
Publication:
[1] L. Xu, H. Liu, J. Song, R. Li, Y. Hu, X. Zhou, P. Patras, TransMUSE: Transferable traffic prediction in multi-service edge networks, Computer Networks (2022).